Goranka Bjedov
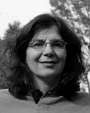
Goranka Bjedov works as a Capacity Planning Engineer at Facebook. Her main interests include performance, capacity and reliability analysis. Prior to joining Facebook, Goranka has also spent five years performance testing at Google and worked for Network Appliance and AT&T Labs. Prior to that she was a professor at Purdue University. A speaker at numerous testing and performance conferences around the world, Goranka has authored many papers, presentations and two textbooks.
Sessions
Performance and Capacity in a Cloud
Friday 11.10 - 12.00 in: Honey Badger
As the software world continues to shift to cloud based solutions, testing professionals are expected to provide answers to the new questions:
How quickly will the system respond?
How many machines (servers, load balancers, switches, etc.) do we need?
What happens when a machine (or a rack, cluster, data-center) fails?
What is the performance cost of a new feature?
Questions such as these have long been labeled, in the software engineering field, as “important,” “difficult,” and “expensive.”
This session will introduce these topics and give examples for services most people are familiar with.
Tags: Cloud Dev Ops Hard Core Test
Future of Testing and Quality
Thursday 10.00 - 10.50 in: Keyboard Cat
If you feel lost and confused, and even ready to give up – do not despair. First – you are not alone. There are many testing professionals struggling with the same issues. Second – change is constant. While you are trying to figure out what you (and your teams) should be doing today, the rate of change is increasing exponentially. Third – things will get worse (before they get better). Even if you are currently offering a product that does not rely on cloud services or is based on open sourced code, there is a start-up somewhere, working out a cheaper solution to your offering. Cloud and open source are here to stay because they provide cheaper and faster way to deploy products and reach customers.
Software services have entered the infinite complexity era – where it is impossible to understand what any single layer does. At the same time, customer expectations have aligned with what is available – while they certainly would not object to higher quality, they are unwilling to pay for it (in numbers large enough to matter), and would strongly object to any delay in shipping or deploying new features. Coincidentally, this change can be seen outside of our field as well.
Think of this talk as a courtesy invitation to the wake of IEEE 829 (829 Standard for Software Test Documentation) – long has it lived and restricted how we did our work. May all the test cases, specifications, plans and procedures rest in peace.
Tags: Test
Performance in a Large Scale Cloud
Monday 8.30 - 16.30 in: Grinding the Crack
This tutorial will focus on what the participants prefer. Any two-three of the following areas regarding Performance in a large scale cloud can be covered with real experiences from Facebook.
Large scale means: more than 1 data center, more than one cluster/data center, more than 10K machines, more than 10 MW of power
What does performance mean: client-side performance is not the topic of this session, server-side, cluster/data-center side (including all comprising pieces) is, reliability, availability and scalability are included
Performance Monitoring: Why - cannot test extensively, need to react/fix problems quickly, Where - every server, every switch, every load balancer, etc., How - no need to save all the data. data != information
Monitoring Part 1 - live demo of dynolog demon: per second data, 300 seconds kept in memory, averages sent to central store, data easily available for collection and analysis
Monitoring Part 2 - Live performance testing: demo instant dyno, principle: control the load on some machines, keep the load at different levels, use existing monitoring to get information
Information: how many users can we serve and at what level, is there a problem, what should be done about it
Smart deployment guards against service/product failures
Performance Analysis - Part 1: find system performance graphs, find what resource is being exhausted, best case scenario - exhausting CPU, memory, disk, network, worst case scenario - system is "latency bound", demo analysis graphs and data for two production systems
Performance Analysis - Part 2: unix based tools: vmstat, isostat, top, ps, strace, php, /proc, several good reference books for optimizing Linux performance, additional tools for analyzing VMs, etc.
Benchmarking: why - because it can identify performance degradation in code as they are made, where - any piece of code that is sensitive to performance or cost, how - many open source tools: JMeter, grinder, FunkLoad, OpenSTA, etc., should run on a nightly basis, results should be analyzed by a human.
Capacity Planning: goal: make sure there is enough capacity to support new features, new users and code degradation, requires planning at least a year ahead, gating factor is (usually) power available